Contextual Content Discovery: You've forgotten about the API endpoints

Contents
- Overview
- What’s wrong with content discovery?
- Content discovery tools over the years
- The lightbulb moment
- Data collection
- Finding APIs worth bruteforcing
- Preliminary results
- How do I use the tool?
- Conclusion
- Assetnote
Overview
Presented at BSides Canberra 2021, slides available: PDF & Keynote (with videos).
As a team, we’re passionate about content discovery as it has historically led to the discovery of vulnerabilities. Typically, these days, content discovery usually involves tools like ffuf with a large wordlist. Over the last ten years, we have seen content discovery tools iterate on many important features (such as filtering and recursion) but a greater focus has been on making these tools faster, not necessarily innovating in the field of content discovery.
Over time, we have also seen a great shift in application development where APIs have become the backbone of server-side functionality. With single page applications taking off and technologies such as Express, Rails, Flask and other API centric frameworks becoming a centerpiece of web applications, we believed that content discovery tooling also needed to evolve to account for this.
Modern API frameworks may require the correct HTTP method, headers, parameters and values in order to produce a valid server-side response (non 404). Our tooling sends requests with context (HTTP methods, headers, parameters and values) by leveraging a large dataset composed of OpenAPI/Swagger specifications from the internet.
When targeting hosts running APIs, this has proven to be an extremely effective method in finding endpoints that typical content discovery tools are not capable of.
Swagger files were collected from a number of datasources, including an internet wide scan for the 20+ most common swagger paths. Other datasources included GitHub via BigQuery, APIs.guru and SwaggerHub.
In order for us to effectively discover content on API based application frameworks, we have developed a tool called Kiterunner and an accompanying datasets: routes-large.kite.tar.gz and routes-small.kite.tar.gz.
If you’re interested in the raw OpenAPI/Swagger files we collected by scanning the internet and from other datasources, you can download them from here. Additionally, if you’re interested in using traditional content discovery tools with our dataset, download swagger-wordlist.txt.
If you would like to learn about how we’ve tackled this problem, continue reading on as we explain the nuances of this problem.
What’s wrong with content discovery?
Content discovery tooling currently relies on static txt files as wordlists and it is up to the user to perform bruteforces using different HTTP methods or to have wordlists with parameters and values pre-filled.
All current tooling operates this way, however, long gone are the days where we are only bruteforcing for static files and folders (legacy web applications). We live in a world where API endpoints account for a large attack surface, and this attack surface can go unnoticed.
We’ve seen a huge shift in how web applications are being built over the last 10 years. Let’s take a look at some examples of API implementations in different frameworks:
Flask
In this example, we can see that there is an API endpoint which requires a path such as <span class="code_single-line">/api/v1/notes/1</span> in order to reach the server-side functionality.
We can also note that this endpoint accepts GET, PUT and DELETE requests, where if the <span class="code_single-line">key</span> integer value is not present in the notes dictionary, a not found exception will be raised.
Unless your wordlist has <span class="code_single-line">/api/v1/notes/<integer></span>, you will miss this API endpoint. Furthermore, if this endpoint only accepted PUT and DELETE requests, you would miss this API endpoint unless you bruteforced content with that specific HTTP method.
With Kiterunner, we leveraged our OpenAPI/Swagger datasets to automatically fill out the correct values for API endpoints, whether they are UUIDs, integers or strings. This gives us a higher chance of reaching API endpoints such as these.
Rails
Similarly, in this example we can see that there are a number of API endpoints that are only reachable by providing the correct <span class="code_single-line">todo_id</span> and in some cases the item <span class="code_single-line">id</span>.
We can see that these endpoints accept POST, PUT and DELETE requests, only if the correct <span class="code_single-line">todo_id</span> and item <span class="code_single-line">id</span> are provided.
Unless your content discovery tool was configured to send POST/PUT/DELETE requests and your wordlist had <span class="code_single-line">/todos/1/items</span> or <span class="code_single-line">/todos/1/items/1</span>, it’s very likely that you will miss these API endpoints.
Express
Most content discovery tools will miss this API endpoint unless there is a path in the wordlist that contains valid values for the parameters <span class="code_single-line">id</span>, <span class="code_single-line">date</span> and <span class="code_single-line">size</span>.
As mentioned earlier, we replace placeholder values in Kiterunner to the best of our ability, based off our OpenAPI/Swagger dataset.
Content discovery tools over the years
As hackers, we’ve invested a lot of time and effort into improving content discovery tooling over the years. Some notable projects include:
All of these tools have done an excellent job at iterating on the concept of content discovery, whether that be speed improvments or usability features.
We pay our respects to all of the tooling built over the years and hope that our work in this area pushes the field of content discovery further.
The lightbulb moment
The primary motivation for us at Assetnote in building Kiterunner and furthering the field of content discovery was driven by our initial idea of leveraging OpenAPI/Swagger specifications for content discovery.
API specifications give us all the information we need about APIs. The correct HTTP methods, headers, parameters and values. This context is essential for so many APIs built in modern frameworks in order to receive a non 404 response.
Our hypothesis was that with enough API specifications collected from the internet, we would be able to compile a dataset that is effective in contextual content discovery. Using this large dataset of OpenAPI/Swagger specifications, we could build a tool that is capable of making requests with the correct context.
We made it our mission to collect as many API specifications as we could from the internet. We created a tool named kitebuilder which converted OpenAPI/Swagger specifications into our own intermediary JSON specification.
The intemediary data can be found below if you would like to try and use it for other projects that require this data:
- routes-large.json.tar.gz (118MB compressed, 2.6GB decompressed)
- routes-small.json.tar.gz (14MB compressed, 228MB decompressed)
From this intemediary format, we were able to then generate a dataset that could be used by our tool Kiterunner.
Data collection
BigQuery
BigQuery is magical.
In order to build our dataset, we leveraged BigQuery’s public datasets. On BigQuery, you can find GitHub’s public dataset, which is updated on a weekly basis. This dataset has terabytes of data, and was a perfect gold mine for us to extract Swagger files from.
This dataset can be accessed through the following URL: https://console.cloud.google.com/bigquery?p=bigquery-public-data&d=github_repos&page=dataset.
First, we select all of the files which have a path which end with either <span class="code_single-line">swagger.json</span>, <span class="code_single-line">openapi.json</span> or <span class="code_single-line">api-docs.json</span> and save it to a new BigQuery table:
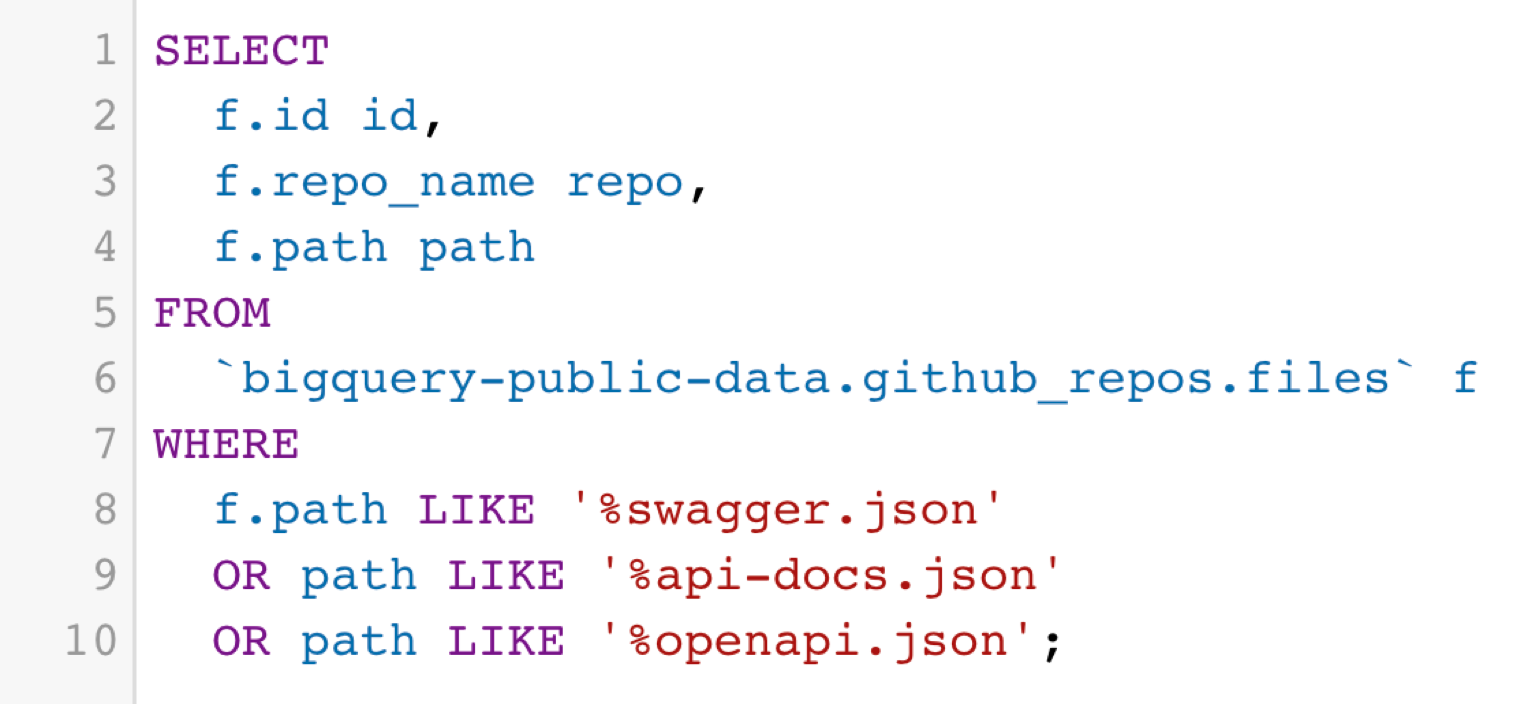
Then we obtain the contents of all of these files, by running the following query:
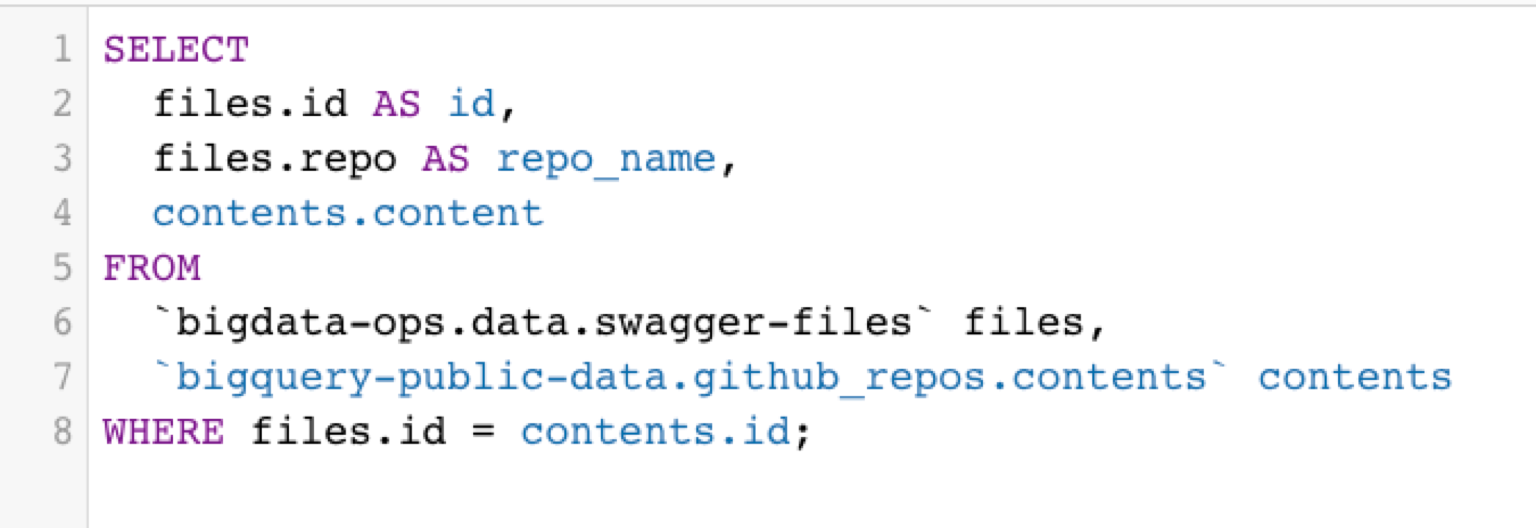
🎉 We now have ~11,000 Swagger files 🎉
APIs.Guru
APIs.Guru is an OpenAPI directory which is essentially a machine readable Wikipedia for REST APIs. The project collects swagger specifications and makes them available in a normalized format. It’s an open source project and all of the data they have collected can be accessed through a REST API.
We were able to obtain all of the Swagger APIs from this API using the following command:
In order to download all the Swagger files to disk, we used the following command:
This was an effective way of downloading all the Swagger files within APIs.Guru as seen in the screenshot below:
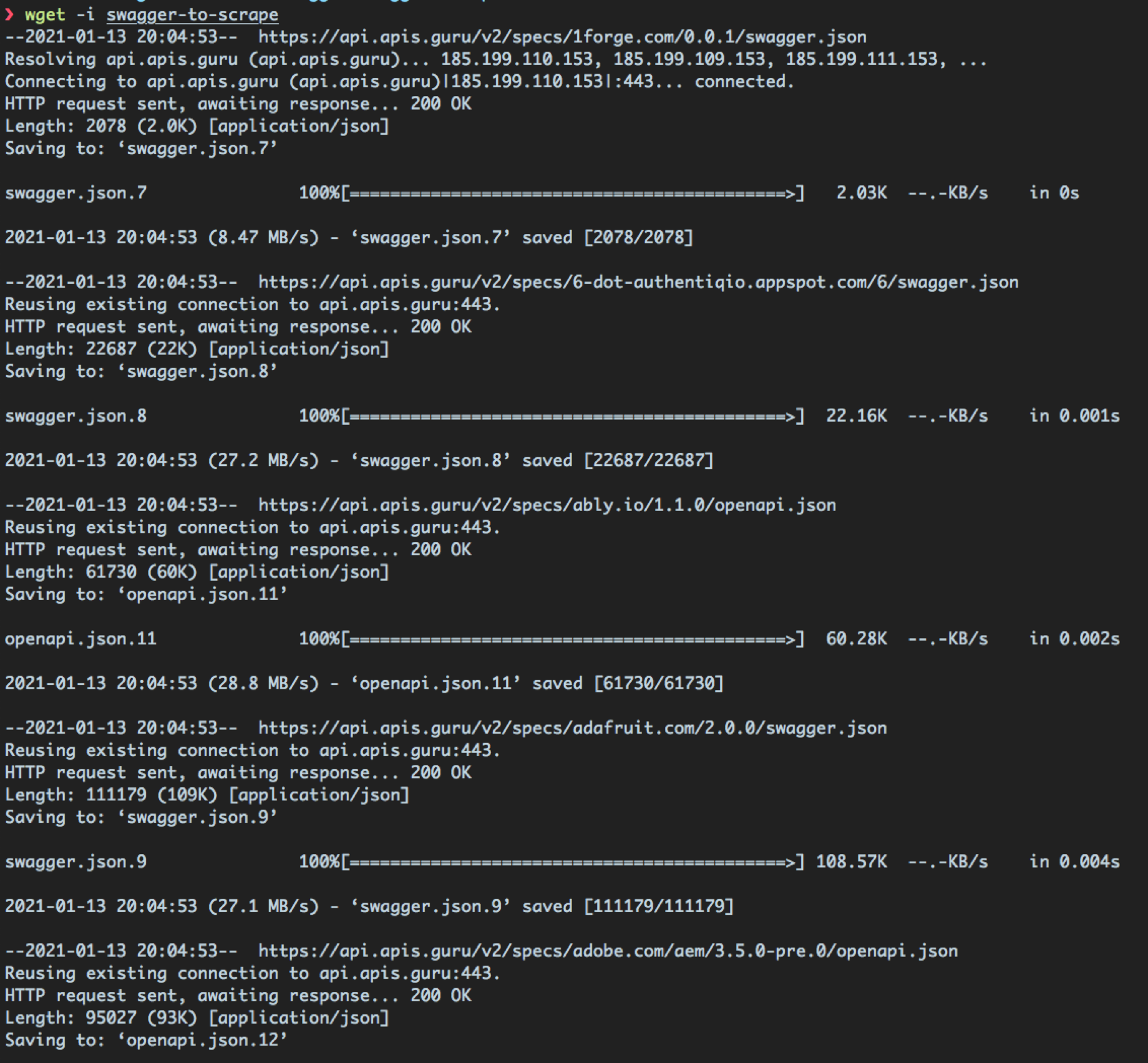
🎉 We now have ~14,000 Swagger files 🎉
SwaggerHub
SwaggerHub is an API design and documentation platform built for teams to drive consistency and discipline across their API development workflow.
By browsing SwaggerHub, you can see that they have a total of 434495 Swagger specifications.
Unfortunately, their API limited us and we were only able to download 10k specifications.
You can find the code we wrote to scrape SwaggerHub here.
🎉 We now have ~23,000 Swagger files 🎉
Scanning the Internet
When scanning the internet, we highly suggest setting up an RDNS record pointing to a web server you control that explains the scanning traffic. This greatly reduces the number of abuse requests.
We scanned the internet for 22 paths that could contain Swagger specifications. The following is a list of paths and the total number of hits we received:
- /swagger/v1/swagger.json - 11173 hits
- /swagger/v1/swagger.yaml - 5048 hits
- /swagger/v2/swagger.json - 5107 hits
- /swagger/v2/swagger.yaml - 4919 hits
- /swagger/v1/api-docs - 5115 hits
- /swagger/v2/api-docs - 5255 hits
- /swagger/api-docs - 6049 hits
- /v2/api-docs - 27189 hits
- /v1/api-docs - 314 hits
- /api-docs - 2454 hits
- /swagger.json - 23983 hits
- /swagger.yaml - 4816 hits
- /api/swagger.json - 5313 hits
- /api/swagger.yaml - 1689 hits
- /api/v1/swagger.json - 1732 hits
- /api/v1/swagger.yaml - 680 hits
- /api/v2/swagger.json - 91101 hits
- /api/v2/swagger.yaml - 404 hits
- /api/docs - 1125 hits
- /api/api-docs - 461 hits
- /static/api/swagger.json - 187 hits
- /static/api/swagger.yaml - 152 hits
We leveraged passive datsources such as Rapid7’s HTTP and HTTPs datasets, combined with an internet wide masscan to fill in the deltas.
Additionally, we only scanned port 80 and 443, focusing on known API documentation paths, scoped to Swagger 2.0 complaint files.
In order to facilitate the scanning, we used Zgrab2, with a custom HTTP module made for Swagger specification files.
Custom tooling written in golang was used to take the 20GB output of zgrab2 and deserialise to individual, validated and deduplicated API specifications.
🎉 We now have ~67,500 Swagger files 🎉
Finding APIs worth bruteforcing

In order to find APIs on the internet, we deployed a large number of API centric frameworks and fingerprinted them. This provides us with a good understanding on what to look for before running Kiterunner over
We’ve created a list of signatures that you can use to discover API endpoints on the internet. We cover the following APIs:
- Adonis
- Aspnet
- Beego
- Cakephp
- Codeigniter
- Django
- Dropwizard
- Echo
- Express
- Fastapi
- Fastify
- Flask
- Generic
- Golang-HTTP
- Hapi
- Jetty
- Kestrel
- Koa
- Kong
- Laravel
- Loopback
- Nest
- Nginx
- Phalcon
- Playframework
- Rails
- Sinatra
- Spark
- Spring-boot
- Symfony
- Tomcat
- Tornado
- Totaljs
- Yii
You can find these signatures here. These signatures contain expected HTTP responses and example Censys queries to discover hosts running these APIs on the internet.
Preliminary Results
After developing the dataset and tooling, we spent some time proving the concept of contextual bruteforcing leading to the discovery of API endpoints that other content discovery tools would struggle finding.
Download API Endpoint
Take for example, the following request:
<span class="code_single-line">GET /download = 404 Not Found</span>
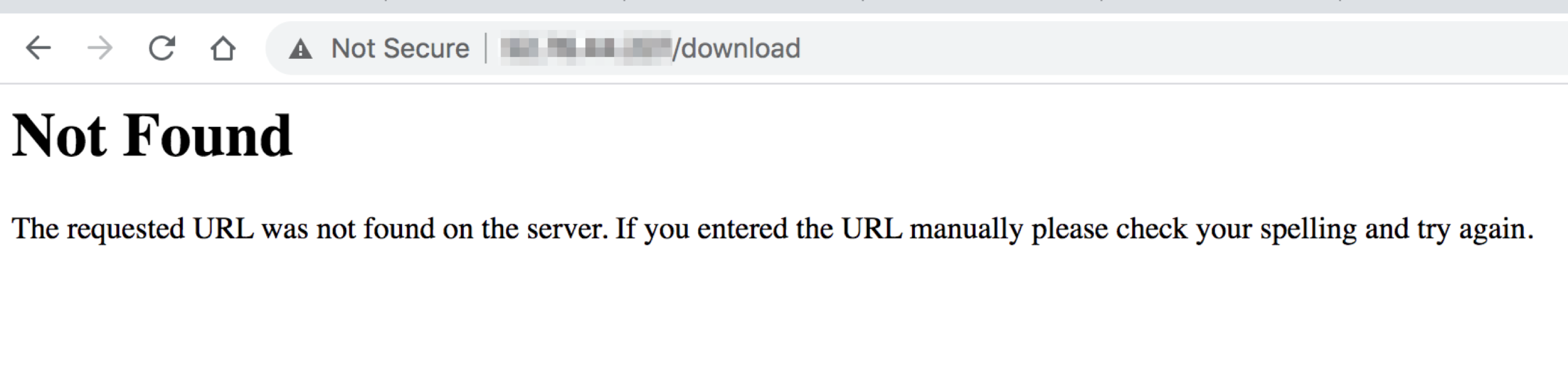
If your wordlist only has <span class="code_single-line">/download</span>, you may think that there is no content. However, with Kiterunner, we saw the following results returned:
<span class="code_single-line">GET /download/17506499 = 200 OK</span>
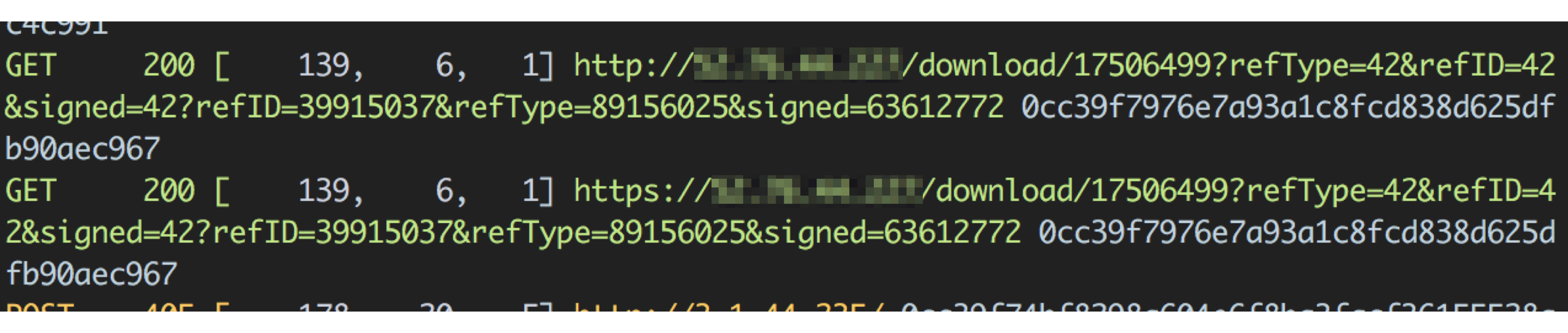
When visiting the fully formed API path <span class="code_single-line">/download/17506499</span>, we saw the following response:

Unless your wordlist had a path like /download/<int>, you would have missed this discovery.
User Create API Endpoint
In this example, it is highly unlikely that any other content discovery tool would have picked up this API endpoint.
The following request returned a 404:
<span class="code_single-line">GET /user/create = 404 Not Found</span>

However, with Kiterunner, we saw the following output:
<span class="code_single-line">POST /user/create = 500 Internal Server Error</span>

GET is 404, POST is 500, indicating that this endpoint does exist.
We were able to replay this request to Burp Suite using the following command:
Which resulting in the following request:
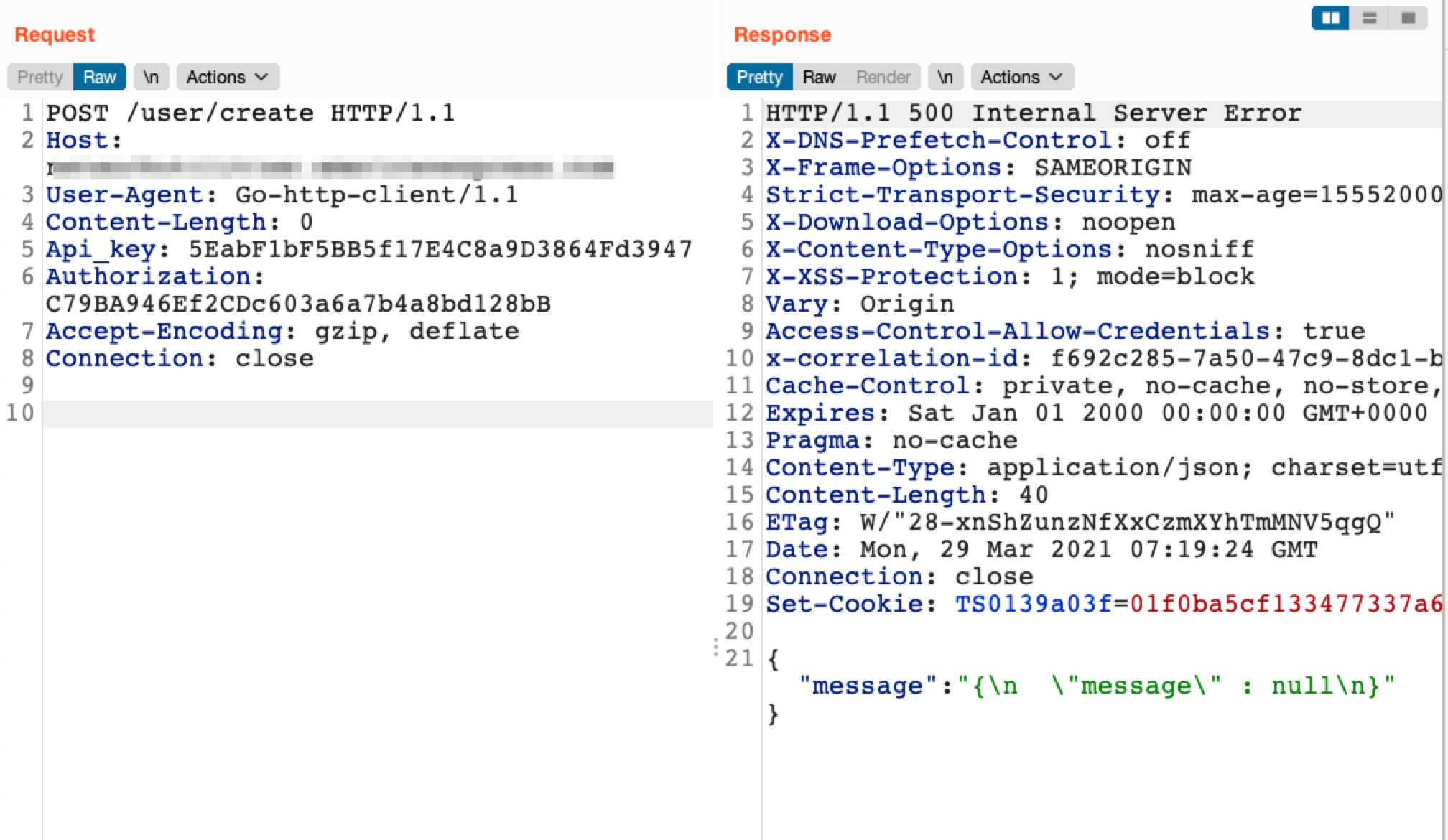
The response indicates that this API endpoint exists however we are likely missing the correct parameters. We were able to use Param Miner to guess the JSON parameters for this endpoint.
Images Endpoint (Local File Read)
Traditional content discovery tools would return the following:
<span class="code_single-line">GET /images = 404 Not Found</span> <span class="code_single-line">GET /images/ = 404 Not Found</span>
However, when using Kiterunner, we noticed that we were given a 301 response status code when making a request with <span class="code_single-line">/images/</span> and having any suffix:

Upon visiting this endpoint, the response returned indicated that it couldn’t locate the image indicated by our suffix path:

We experimented with this and found that it was actual a local file read vulnerability. We could request the following path to leak the contents of the <span class="code_single-line">/etc/passwd</span> file:
<span class="code_single-line">GET /secured/28572478/images//etc/passwd = 200 OK (with file contents)</span>

How do I use the tool?
Download a release for your operating system from the releases page: github.com/assetnote/kiterunner/releases
Contextual Bruteforcing
Download the dataset routes-large.kite.tar.gz (40MB compressed, 577MB decompressed) or the smaller dataset routes-small.kite.tar.gz (2MB compressed, 35MB decompressed).
After downloading the tool and dataset, Kiterunner can be used through a command like the following:
Put the hosts you wish to scan with Kiterunner inside a single text file, one host per line.
The following options are available for the <span class="code_single-line">kr scan</span> (contextual bruteforcing):
Vanilla Bruteforcing
It’s also possible to use Kiterunner as a traditional content discovery tool (txt file wordlists). Kiterunner has built in support for Assetnote Wordlists.
Run the following command to list Assetnote Wordlists:
This will return a table of contents like below:
Use your favourite txt wordlist with Kiterunner, combined with the <span class="code_single-line">apiroutes-210228</span> Assetnote Wordlist through the following command:
The following additional options are available for the <span class="code_single-line">kr brute</span> (vanilla bruteforcing):
Replaying requests
When you receive a bunch of output from kiterunner, it may be difficult to immediately understand why a request is causing a specific response code/length. Kiterunner offers a method of rebuilding the request from the wordlists used including all the header and body parameters.
- You can replay a request by copy pasting the full response output into the kb <span class="code_single-line">replay</span> command.
- You can specify <span class="code_single-line">a --proxy</span> to forward your requests through, so you can modify/repeat/intercept the request using 3rd party tools if you wish
- The golang net/http client will perform a few additional changes to your request due to how the default golang spec implementation (unfortunately).
Conclusion
Content discovery on API hosts requires a different approach in order to achieve maximum coverage. We were able to operationalize OpenAPI/Swagger specifications from a number of datasources to assist in the discovery of API endpoints from a black-box perspective.
From the preliminary results gathered using Kiterunner, we found numerous examples where current content discovery tooling would have failed to pick up endpoints which represented legitimate attack surface.
By taking a contextual approach at discovering endpoints (correct HTTP method, headers, parameters and values), we were able to find content that would have been tricky to pick up otherwise.
Most of our challenges were related to the engineering efforts required to deal with OpenAPI/Swagger specifications from arbitrary sources and making Kiterunner a performant bruteforcer. We plan on writing a separate blog post to go over the engineering challenges faced during this security research.
Next time you’re bruteforcing an application with an API, consider using Kiterunner alongside your other content discovery workflows.
Assetnote
We love content discovery at Assetnote. Working on research and engineering problems surrounding this problem area has always been exciting to us.
Assetnote Continuous Security automatically maps your external assets and monitors them for changes and security issues to help prevent serious breaches. If you want to protect your attack surface and would like a demonstration of our product, please reach out to us by submitting our contact form.
On a final note, Assetnote is hiring across a number of engineering, security and operations roles. One of the best parts of working at Assetnote is the ability to combine interesting engineering challenges with security research as part of the every day work on our products. If you are interested in joining our team and working on cutting edge security products check out our careers page.
Credits
The following people were involved in this research:
More Like This
Ready to get started?
Get on a call with our team and learn how Assetnote can change the way you secure your attack surface. We'll set you up with a trial instance so you can see the impact for yourself.